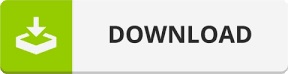

This, however, implies concessions to conciliate the original conceptual representation of data with the data model required by the framework (eg, The European Hospital George Pompidou HDW ). Many solutions also implement generic frameworks, such as Informatics for Integrating Biology and the Bedside (i2b2) database. The Enterprise Data Trust relies heavily on industrial solutions to cope with the huge amount of data. This is, nevertheless, particularly important in an IR context, as potential clinical questions and inquiries from health professionals are formulated in terms of their vision of the conceptual organization of data that derive from the actual patient management process.
TABLE INDEX INDEX INCLUSION POWERDESIGNER FULL
Furthermore, they do not necessarily allow the full and independent visualization and retrieval of the different atomic entities conceptually composing the whole scope of clinical information (eg, Stanford Translational Research Integrated Database Environment or STRIDE and Data Warehouse for Translational Research or DW4TR ). From a holistic point of view, the majority of these solutions provide aggregated data mainly focusing on patient data as a result. This kind of data repository centralizes clinical, demographic, and administrative data within a uniform and consistent data model. An HDW is defined as a grouping of data from diverse sources accessible by a single data management system. A common approach to information retrieval (IR) in clinical unstructured text outside the basic full-text search comprises partially restructuring the original texts using semantic annotators (eg, MetaMap ) that map words or expressions to concepts from domain knowledge databases.Ĭonsistently aggregating all these scattered, big, complex, and diversely structured data is, in fact, the role of health data warehouses (HDWs). The background knowledge, as represented in terminologies and ontologies (T&Os that describe the domain), plays a crucial role in any clinical NLP task.

To process unstructured data, the main approaches rely on natural language processing (NLP) methods. However, in the study by Raghavan et al, the authors found that not only unstructured data were essential to resolve between 59% and 77% of some clinical trials criteria but also that combining the use of structured and unstructured data enabled leverage of patient recruitment. This unstructured information is particularly relevant in the context of cohort selection tasks. Moreover, the health data produced are of different nature some data are natively structured (eg, diagnosis-related group codings and laboratory tests results), but an important part of medical information remains in unstructured free-text clinical narratives (CNs eg, admission notes, history and physical reports, discharge summaries, radiology reports, and pathology reports). For instance, according to research, in the United States, the health care system alone reached 150 exabytes (1.5×10 20 bytes) in 2011 and will reach the yottabyte scale (10 24 bytes) in the near future. Health data can synthetically and legitimately be described as big data. Second, the significant amount of data generated results in problematic management of data both in terms of data storage capabilities and data access performances. First, the data are produced and maintained by different systems and health professionals and are consequently spread over multiple sources and even across multiple establishments. However, the exploitation of these data remains difficult for several reasons. Hospitals maintain important health data that can be used in various contexts: first and foremost, clinical care and then data reusability, clinical decision support systems, clinical research and cohort selection, education, and indicators.
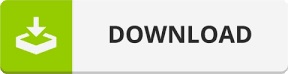